This week, progress on the new feature work has been limited (though I did manage to complete some new features). On the one hand, there weren’t many features left to implement. On the other hand, I discovered some bugs from previous work this week and focused on fixing them.
The first issue was related to querying and displaying the history table for each fileset. Ideally, for example, if we merge fileset 2 into fileset 23, and then fileset 23 into fileset 123, the history page for fileset 123 should trace back to which filesets it was merged from. Initially, my code simply used SELECT * FROM history WHERE fileset = {id} OR oldfileset = {id}
, which was clearly insufficient.
So, I initially thought about using a method similar to an unoptimized union-find (a habit from competitive programming 🤣) to quickly query merged filesets. However, I realized this would require an additional table (or file) to store the parent nodes of each node in the union-find structure, which seemed cumbersome. Therefore, I opted to straightforwardly write a recursive query function, which surprisingly performed well, and I used this naive method to solve the problem.
Another issue arose on the manual match page for each fileset. Although the program always identifies the fileset with the most matches, I found that the number of matched files between two filesets was significantly off during testing. Upon investigation, I discovered that the displayed count was consistently five times the actual number of matches. Where did this factor of five come from? Initially, I was baffled.
After logging several entries, I finally pinpointed the problem. The manual match page retrieves data from the database, while the dat_parser
uses data from DAT files. These sources store file data in slightly different formats. For example, the format from a DAT file might look like:
[{"name":"aaa", "size":123, "md5":"123456", "md5-5000":"45678"}, {"name":"bbb", "size":222, "md5":"12345678", "md5-5000":"4567890"}...]
But the format retrieved from the database might look like:
[{"name":"aaa", "size":123, "md5":"123456"}, {"name":"aaa", "size":123, "md5-5000":"45678"}...]
As a result, it became clear that scan-type filesets stored in the database had five types of file_checksum
(meaning each file was treated as five separate files when matched), leading the counter to naturally increase by five for each such file encountered. After simply reorganizing the retrieved database data, I resolved this bug.
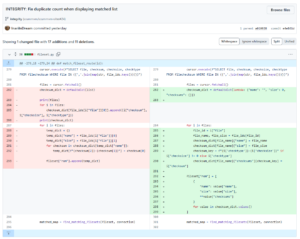